Finding comfort through machine learning
A recent study leverages conflicting information provided by building occupants when answering multiple related questions about their thermal comfort to find the real “comfort zone” for most people in a building.
People who have worked in a large building have likely encountered the experience of being too hot or too cold in their work space. Regulating temperature in such buildings is essential to both ensuring comfort of those using the space, as well as maximizing the building’s energy efficiency.
Heating, ventilation, and air conditioning (HVAC) systems often struggle to balance these needs, but machine learning (ML) models can be helpful in predicting how people feel about temperature in different areas of a building to improve efficiency. While these models can speak to the complex relationship between the physical environment and the occupants’ subjective thermal perceptions, the models can also present issues, such as biased human-perception data and uncertainties that can lead to inaccurate predictions and inefficient control of the building.
In order to improve this ML approach, a team of researchers from the Civil and Environmental Engineering Department and Carnegie Mellon University has proposed a method that combines data and models, using Multidimensional Association Rule Mining (M-ARM) to find and correct biases in human responses to temperature.
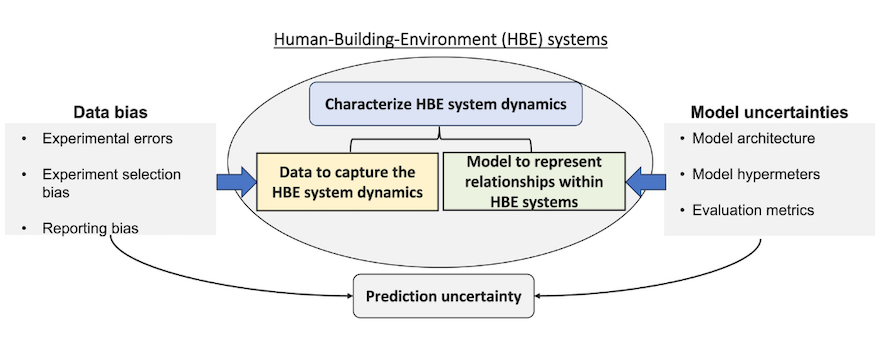
Uncertainty sources for characterizing the Human–Building–Environment (HBE) system dynamics.
In research featured in Building and Environment, they tested this method on seven ML models and found that it improves the accuracy of predicting how people will feel about the temperature. The research leverages conflicting information provided by building occupants when answering multiple related questions about their thermal comfort to find the real “comfort zone” for most people in a building. The study analyzed the miscalibration issues associated with current methods and identified potential instances of subjective data biases using M-ARM.
“This work has the potential to contribute to saving energy, without having many people who use these large buildings complaining of discomfort,” said Pingbo Tang, associate professor of civil and environmental engineering, who led the study.
This work has the potential to contribute to saving energy, without having many people who use these large buildings complaining of discomfort.
Pingbo Tang, Associate Professor, Civil and Environmental Engineering
Tang notes that defective data sets that are currently being used are potentially resulting in excess energy consumption. While human comfort is based on dimensions such as humidity and temperature, clothing also plays a part.
“There are more factors than just a person’s perception of the temperature,” says Tang. “This work is about using the question-answering behavior of the person when they are facing a few related thermal comfort questions to adjust self-conflicts and estimate reality.”
Considering impact factors such as size of the dataset, different types of classifiers, and calibration methods, the authors found that they can significantly improve prediction reliability and reduce errors in the models currently used.
The results of this study provide insights into the advancement of ML-based strategies, with the goal of achieving more reliable thermal perception predictions. This work could lead to better strategies for controlling temperature in buildings to make occupants more comfortable and reduce energy consumption.