Training robotic arms with a hands-off approach
Researchers at Carnegie Mellon University recently trained a robotic arm with human movements generated by artificial intelligence.
Humans often take their fine motor abilities for granted. Recreating the mechanical precision of the human body is no easy task—one that graduate students in CMU’s Mechanical Engineering Department hope to simplify through artificial intelligence.
Ph.D. candidates Alison Bartch and Abraham George, under the guidance of Amir Barati Farimani, assistant professor of mechanical engineering, first recreated a simple task, such as picking up a block, using a virtual reality simulation. Using this example, they then were able to augment different “human-like” examples of the movements to aid the robot’s learning.
“If I want to show you how to do a task, I just have to do it once or twice before you pick up on it,” George said. “So it’s very promising that now we can get a robot to replicate our actions after just one or two demos. We have created a control structure where it can watch us, extract what it needs to know, and then perform that action.”
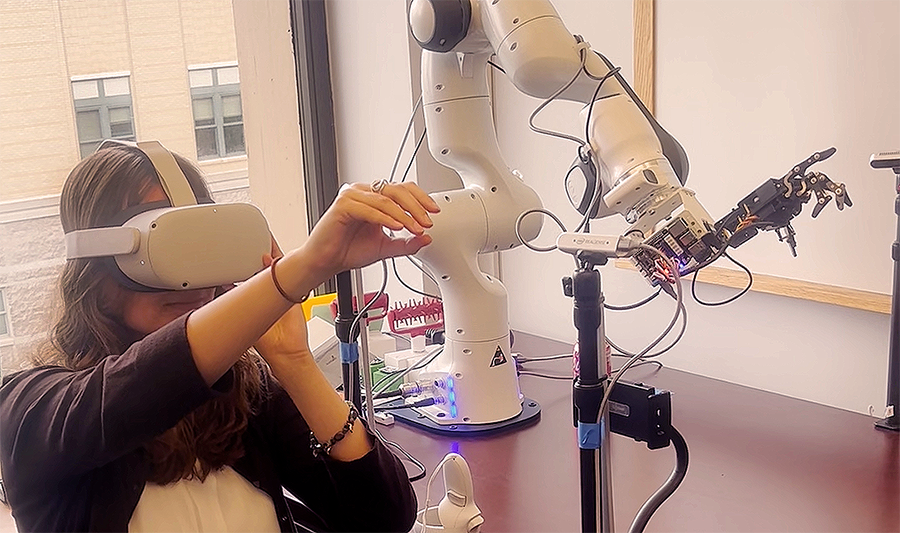
The team found that the augmented examples helped to significantly decrease the robot’s learning time for the block pick-and-place task compared to a machine learning architecture alone. This, paired with the collection of human data through a VR headset simulation, means this research method has the potential to produce promising results with “under a minute of human input.”
George described the challenge of creating augmented examples that were both reliable and “novel” for the AI to learn from, so that it could recognize more nuanced differences in the same movements.
“A good analogy for this is when you are trying to train a computer to recognize a picture of a dog, you might show it hundreds of images of dogs versus cats.” George said. “But we are trying to train the computer to identify a dog based on just one augmented image. So if we show it a different breed of dog, the computer is going to struggle to identify it as a dog.”
We have created a control structure where it can watch us, extract what it needs to know, and then perform that action.
Abraham George, Ph.D. candidate, Mechanical Engineering
Already looking forward, Bartch plans to use similar methods to teach the robot how to interact with more malleable material—particularly clay—and predict how it will shape them.
“For the end goal of having robots in the world, they need to be able to predict how different materials are going to behave,” Bartch said. “If you think about an assistant robot at home, the materials they will interact with are deformable: food is deformable, sponges are deformable, clothes are deformable.”
This research was presented at the 2023 International Conference on Robotics and Automation.